Wallaroo Edge Deployment & Inference for U-Net Computer Vision for Brain Segmentation
Features:
Models:
This tutorial and the assets can be downloaded as part of the Wallaroo Tutorials repository.
U-Net for Brain Segmentation Deployment in Wallaroo
The following example uses the U-Net for brain segmentation model trained to detect lower-grade gliomas to demonstrate how to:
- Deploy the model into a Wallaroo Ops server.
- Perform a sample inferences via the Wallaroo SDK and the API calls.
- Publish the pipeline to an OCI (Open Container Initiative) registry service.
- Deploy the published pipeline to an edge device as a Wallaroo Inference Server, and perform the same inference calls.
Prerequisites
- A Wallaroo Community Edition or Enterprise Edition server with Edge Deployment enabled.
- For a free license of Wallaroo Community Edition, go to https://portal.wallaroo.community/.
- A x64 edge device capable of running Docker
References
- Edge Deployment Registry Guide
- Brain MRI segmentation: The original evaluation and training images. These can be used with this inference server.
Tutorial Steps
Import Libraries
The first step is to import the libraries we’ll be using. These are included by default in the Wallaroo instance’s JupyterHub service.
Verify that the following Python libraries are installed. The wallaroo
library is included with the Wallaroo Ops JupyterHub instance.
pillow
torchvision
pandas
pyarrow
wallaroo
References
import wallaroo
from wallaroo.pipeline import Pipeline
from wallaroo.deployment_config import DeploymentConfigBuilder
from wallaroo.framework import Framework
# used to convert the Image into a numpy array
from PIL import Image
from torchvision import transforms
import pyarrow as pa
import numpy as np
import pandas as pd
import requests
# used to display dataframe information without truncating
from IPython.display import display
pd.set_option('display.max_colwidth', None)
pd.set_option('display.max_columns', None)
Connect to the Wallaroo Instance
The first step is to connect to Wallaroo through the Wallaroo client. The Python library is included in the Wallaroo install and available through the Jupyter Hub interface provided with your Wallaroo environment.
This is accomplished using the wallaroo.Client()
command, which provides a URL to grant the SDK permission to your specific Wallaroo environment. When displayed, enter the URL into a browser and confirm permissions. Store the connection into a variable that can be referenced later.
If logging into the Wallaroo instance through the internal JupyterHub service, use wl = wallaroo.Client()
. For more information on Wallaroo Client settings, see the Client Connection guide.
The option request_timeout
provides additional time for the Wallaroo model upload process to complete.
wl = wallaroo.Client()
Create Retrieve WOrkspace and Pipeline
We retrieve the workspace and pipeline used to create the pipeline publish.
The workspace will then be set as the Current Workspace. Model uploads and pipeline creation through the SDK are set in the current workspace.
workspace_name = "unet-detection-run-anywhere-demonstration"
model_name = "pt-unet"
model_file_name = './models/unet.pt'
pipeline_name = "pt-unet"
workspace = wl.get_workspace(name=workspace_name, create_if_not_exist=True)
wl.set_current_workspace(workspace)
pipeline = wl.get_pipeline(pipeline_name)
Retrieve Pipeline Publish
We retrieve the pipeline publish details via the pipeline.publishes() method. We assume that this pipeline only has the one publish created from the previous tutorial run, and display it’s details that include the Docker Run command we will use for the deployment step.
pipeline.publishes()
id | pipeline_version_name | engine_url | pipeline_url | created_by | created_at | updated_at |
---|---|---|---|---|---|---|
1 | 18a2e65e-d600-471f-80fe-6b60030f9dda | ghcr.io/wallaroolabs/doc-samples/engines/proxy/wallaroo/ghcr.io/wallaroolabs/fitzroy-mini:v2024.4.0-6077 | ghcr.io/wallaroolabs/doc-samples/pipelines/pt-unet:18a2e65e-d600-471f-80fe-6b60030f9dda | john.hansarick@wallaroo.ai | 2025-29-Apr 18:51:29 | 2025-29-Apr 18:51:29 |
pipeline.publishes()[0]
ID | 1 | |
Pipeline Name | pt-unet | |
Pipeline Version | 18a2e65e-d600-471f-80fe-6b60030f9dda | |
Status | Published | |
Engine URL | ghcr.io/wallaroolabs/doc-samples/engines/proxy/wallaroo/ghcr.io/wallaroolabs/fitzroy-mini:v2024.4.0-6077 | |
Pipeline URL | ghcr.io/wallaroolabs/doc-samples/pipelines/pt-unet:18a2e65e-d600-471f-80fe-6b60030f9dda | |
Helm Chart URL | oci://ghcr.io/wallaroolabs/doc-samples/charts/pt-unet | |
Helm Chart Reference | ghcr.io/wallaroolabs/doc-samples/charts@sha256:ec23c28cc1427076bdab1566b45348f68cc7c46351d5aa49a35c58ee79eec2a7 | |
Helm Chart Version | 0.0.1-18a2e65e-d600-471f-80fe-6b60030f9dda | |
Engine Config | {'engine': {'resources': {'limits': {'cpu': 4.0, 'memory': '3Gi'}, 'requests': {'cpu': 4.0, 'memory': '3Gi'}, 'arch': 'x86', 'accel': 'none', 'gpu': False}}, 'engineAux': {'autoscale': {'type': 'none'}}} | |
User Images | [] | |
Created By | john.hansarick@wallaroo.ai | |
Created At | 2025-04-29 18:51:29.081389+00:00 | |
Updated At | 2025-04-29 18:51:29.081389+00:00 | |
Replaces | ||
Docker Run Command |
Note: Please set the EDGE_PORT , OCI_USERNAME , and OCI_PASSWORD environment variables. | |
Helm Install Command |
Note: Please set the HELM_INSTALL_NAME , HELM_INSTALL_NAMESPACE ,
OCI_USERNAME , and OCI_PASSWORD environment variables. |
pipeline.publishes()[0].pipeline_url
'ghcr.io/wallaroolabs/doc-samples/pipelines/pt-unet:18a2e65e-d600-471f-80fe-6b60030f9dda'
DevOps - Pipeline Edge Deployment
Once a pipeline is deployed to the Edge Registry service, it can be deployed in environments such as Docker, Kubernetes, or similar container running services by a DevOps engineer.
Docker Deployment
First, the DevOps engineer must authenticate to the same OCI Registry service used for the Wallaroo Edge Deployment registry.
For more details, check with the documentation on your artifact service. The following are provided for the three major cloud services:
- Set up authentication for Docker
- Authenticate with an Azure container registry
- Authenticating Amazon ECR Repositories for Docker CLI with Credential Helper
For the deployment, the engine URL is specified with the following environmental variables:
DEBUG
(true|false): Whether to include debug output.OCI_REGISTRY
: The URL of the registry service.CONFIG_CPUS
: The number of CPUs to use.OCI_USERNAME
: The edge registry username.OCI_PASSWORD
: The edge registry password or token.PIPELINE_URL
: The published pipeline URL.
Docker Deployment Example
Using our sample environment, here’s sample deployment using Docker.
For docker run
commands, the persistent volume for storing session data is stored with -v ./data:/persist
. Updated as required for your deployments.
# create docker run
docker_command = f'''
docker run \
-p $EDGE_PORT:8080 \\
-e OCI_USERNAME=$OCI_USERNAME \\
-e OCI_PASSWORD=$OCI_PASSWORD \\
-e CONFIG_CPUS=4 --cpus=4.0 --memory=3g \\
-e PIPELINE_URL={pipeline.publishes()[0].pipeline_url} \\
ghcr.io/wallaroolabs/doc-samples/engines/proxy/wallaroo/ghcr.io/wallaroolabs/fitzroy-mini:v2024.4.0-6077
'''
print(docker_command)
docker run -p $EDGE_PORT:8080 \
-e OCI_USERNAME=$OCI_USERNAME \
-e OCI_PASSWORD=$OCI_PASSWORD \
-e CONFIG_CPUS=4 --cpus=4.0 --memory=3g \
-e PIPELINE_URL=ghcr.io/wallaroolabs/doc-samples/pipelines/pt-unet:18a2e65e-d600-471f-80fe-6b60030f9dda \
ghcr.io/wallaroolabs/doc-samples/engines/proxy/wallaroo/ghcr.io/wallaroolabs/fitzroy-mini:v2024.4.0-6077
Edge Deployed Pipeline API Endpoints
Once deployed, we can check the pipelines and models available. We’ll use a curl
command, but any HTTP based request will work the same way.
The endpoint /pipelines
returns:
- id (String): The name of the pipeline.
- status (String): The status as either
Running
, orError
if there are any issues.
For this example, the deployment is made on a machine called testboy.local
. Replace this URL with the URL of your edge deployment.
deploy_url = 'http://testboy.local:8081/pipelines'
response = requests.get(
deploy_url
)
display(response.json())
{'pipelines': [{'id': 'pt-unet',
'version': '18a2e65e-d600-471f-80fe-6b60030f9dda',
'status': 'Running'}]}
Edge Inference Endpoint
The inference endpoint takes the following pattern:
/infer
Wallaroo inference endpoint URLs accept the following data inputs through the Content-Type
header:
Content-Type: application/vnd.apache.arrow.file
: For Apache Arrow tables.Content-Type: application/json; format=pandas-records
: For pandas DataFrame in record format.
Once deployed, we can perform an inference through the deployment URL.
The endpoint returns Content-Type: application/json; format=pandas-records
by default with the following fields:
time (Integer): The time since UNIX epoch.
in: The original input data. Returns
null
if the input may be too long for a proper return.out (List): The outputs of the inference result separated by the model’s output fields.
check_failures (List[Integer]): Whether any validation checks were triggered. For more information, see Wallaroo SDK Essentials Guide: Pipeline Management: Anomaly Testing.
metadata (String): The metadata including the model name, etc.
For this example, we will use the same DataFrame with the image data and perform the same API inference request, this time through the edge device located at hostname testboy.local
. Adjust the URL according to your edge deployment.
input_image = Image.open("./data/TCGA_CS_4944.png")
display(input_image)
# preprocess
m, s = np.mean(input_image, axis=(0, 1)), np.std(input_image, axis=(0, 1))
preprocess = transforms.Compose([
transforms.ToTensor(),
transforms.Normalize(mean=m, std=s),
])
input_tensor = preprocess(input_image)
input_batch = input_tensor.unsqueeze(0)
nimage = input_batch.detach().numpy()
nimage.shape
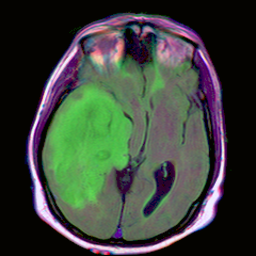
(1, 3, 256, 256)
nimage = input_tensor.detach().numpy()
input_data = {
"input": [nimage]
}
dataframe = pd.DataFrame(input_data)
headers = {
'Content-Type': 'application/json; format=pandas-records'
}
#
deploy_url = 'http://testboy.local:8081/infer'
response = requests.post(
deploy_url,
headers=headers,
data=dataframe.to_json(orient="records")
)
display(pd.DataFrame(response.json()).loc[0, 'out']['output'][0:5])
[1.475215e-05, 1.463294e-05, 1.3977289e-05, 1.3887882e-05, 1.4543533e-05]
Undeploy Edge Pipeline
To undeploy the edge pipeline, either use Control-C
from the terminal. Or, use:
docker ps
CONTAINER ID IMAGE COMMAND CREATED STATUS PORTS NAMES
690f24d4d9b1 ghcr.io/wallaroolabs/doc-samples/engines/proxy/wallaroo/ghcr.io/wallaroolabs/fitzroy-mini:v2024.4.0-6077 "/usr/bin/tini -- /s…" 2 seconds ago Up 1 second 0.0.0.0:8081->8080/tcp, :::8081->8080/tcp nifty_spence
Find the Docker container running. This example we can see the. `wallaroolabs` image running, and give it the kill command from the container id. When the image is no longer available with `docker ps`, the edge deployment of Wallaroo Inference Server has stopped.
```bash
docker stop 690f24d4d9b1
docker ps
CONTAINER ID IMAGE COMMAND CREATED STATUS PORTS NAMES