Wallaroo Frequently Asked Questions
Table of Contents
How Do I Install Wallaroo?
The Wallaroo Install Guides contain all steps on how to register with Wallaroo and receive a license, install Wallaroo into a Kubernetes cluster, and get Wallaroo running.
What Are The Prerequisites For Installing Wallaroo In A Cloud Service?
Wallaroo Community and Wallaroo Enterprise can be installed into a Kubernetes environment with the following requirements:
- Minimum number of nodes: 4
- Minimum Number of CPU Cores: 8
- Minimum RAM: 16 GB
- Kubernetes requirements:
- Kubernetes Version
1.20
is the minimum requirement1.22
is preferred version for Wallaroo versions before 2022.4.1.23
:- Is the preferred version for Wallaroo version 2022.4.
1.23 and above
:- Are the preferred versions for Wallaroo versions 2023.1 and later.
- Are not supported for versions of Wallaroo released before Wallaroo version 2022.4.
1.29
:- The preferred version for Wallaroo 2024.1
- Runtime: containerd is required.
- Kubernetes Version
The following guide demonstrate how to set up a minimum Kubernetes environment in their cloud services and install Wallaroo:
Who Do I Contact For More Help With Wallaroo Community?
Wallaroo Community users can message community@wallaroo.ai for more assistance. Feel free to ask any questions about:
- Installing Wallaroo
- Uploading models and deploying pipelines
- How to use the SDK
How Do I Set Up My Wallaroo Community User Account?
Setting up a Wallaroo Community account is fast and easy at https://portal.wallaroo.community. See the Wallaroo Install Guides for full details on registering an account and downloading your Wallaroo Community License.
How Do I Invite Collaborators And Peers To Work With After Installing Wallaroo?
Yes you can! Wallaroo Community supports up to 2 team members, while Wallaroo Enterprise has no such limitations.
See the Wallaroo User Management Guide for how to add users to your Wallaroo instance. For details on how to add users to a Wallaroo Workspace, see the Wallaroo Workspace Management Guide.
What Are The Resources I Need To Be Able To Learn About The Wallaroo Platform?
Wallaroo has provided the following resources to help you:
- The Wallaroo 101 Guide teaches the basic concepts of how Wallaroo works, then provides a full tutorial for uploading a model and running an inference.
- Wallaroo Tutorials are paired with the Wallaroo Tutorials Repository to provide Jupyter Notebooks that you can upload to Wallaroo along with sample models and data to see how to deploy your own ML Models.
- The Wallaroo Essential SDK walks you the process of user, model, workspace, and pipeline management using the Wallaroo SDK.
How Fast is Wallaroo?
Not only is Wallaroo fast - its more cost efficient. Using the Aloha Tutorial as a benchmark, Wallaroo provides the following:
# inferences per second | inferences/dollar | |
---|---|---|
Vertex | 1.824 | 6,493,506 |
Databricks | 1.856 | 16,000,000 |
SageMaker | 5.008 | 28,169,014 |
Wallaroo | 21.584 | 127,659,574 |
IMPORTANT NOTE
Based on results from ALOHA (ALOHA: Auxiliary Loss Optimization for Hypothesis Augmentation) model, a complex, open-source model used for benchmark and performance testing. Test involved scoring 2 Billion events a day (23k events every second), analyzed in batches running on 16-CPU server on GCP (Vertex), AWS (sageMaker) and Azure (Databricks).How Can I Bring My Models Into Wallaroo?
Absolutely! Wallaroo supports the ONNX ML Standard and can convert other models through our auto-conversion feature.
Supported Models
The following frameworks are supported. Frameworks fall under either Wallaroo Native Runtimes or Wallaroo Containerized Runtimes in the Wallaroo engine. For more details, see the specific framework what runtime a specific model framework runs in.
IMPORTANT NOTE
Verify that the input types match the specified inputs, especially for Containerized Wallaroo Runtimes. For example, if the input is listed as apyarrow.float32()
, submitting a pyarrow.float64()
may cause an error.The Wallaroo Model Runtime is displayed after a model is uploaded with the wallaroo.model.config().runtime()
method. The following table displays the type of Runtime associated with each possible display.
Runtime Display | Model Runtime Space | Pipeline Configuration |
---|---|---|
tensorflow | Native | Native Runtime Configuration Methods |
onnx | Native | Native Runtime Configuration Methods |
python | Native | Native Runtime Configuration Methods |
mlflow | Containerized | Containerized Runtime Deployment |
flight | Containerized | Containerized Runtime Deployment |
Please note the following.
IMPORTANT NOTICE: FRAMEWORK VERSIONS
The supported frameworks include the specific version of the model framework supported by Wallaroo. It is highly recommended to verify that models uploaded to Wallaroo meet the library and version requirements to ensure proper functioning.Native Model Runtimes
Wallaroo ONNX Requirements
Wallaroo natively supports Open Neural Network Exchange (ONNX) models into the Wallaroo engine.
Parameter | Description |
---|---|
Web Site | https://onnx.ai/ |
Supported Libraries | See table below. |
Framework | Framework.ONNX aka onnx |
Runtime | Native aka onnx |
Supported Versions | 1.12.1 |
The following ONNX versions models are supported:
ONNX Version | ONNX IR Version | ONNX OPset Version | ONNX ML Opset Version |
---|---|---|---|
1.12.1 | 8 | 17 | 3 |
- If converting another ML Model to ONNX (PyTorch, XGBoost, etc) using the onnxconverter-common library, the supported
DEFAULT_OPSET_NUMBER
is 17.
Using different versions or settings outside of these specifications may result in inference issues and other unexpected behavior.
ONNX models always run in the Wallaroo Native Runtime space.
Parameter | Description |
---|---|
Web Site | https://www.tensorflow.org/ |
Supported Libraries | tensorflow==2.9.3 |
Framework | Framework.TENSORFLOW aka tensorflow |
Supported File Types | SavedModel format as .zip file |
IMPORTANT NOTE
These requirements are not for Tensorflow Keras models, only for non-Keras Tensorflow models in the SavedModel format. For Tensorflow Keras deployment in Wallaroo, see the Tensorflow Keras requirements.TensorFlow File Format
TensorFlow models are .zip file of the SavedModel format. For example, the Aloha sample TensorFlow model is stored in the directory alohacnnlstm
:
├── saved_model.pb
└── variables
├── variables.data-00000-of-00002
├── variables.data-00001-of-00002
└── variables.index
This is compressed into the .zip file alohacnnlstm.zip
with the following command:
zip -r alohacnnlstm.zip alohacnnlstm/
ML models that meet the Tensorflow and SavedModel format will run as Wallaroo Native runtimes by default.
See the SavedModel guide for full details.
Parameter | Description |
---|---|
Web Site | https://www.python.org/ |
Supported Libraries | python==3.8 |
Framework | Framework.PYTHON aka python |
Python models uploaded to Wallaroo are executed Wallaroo Containerized Runtime.
Note that Python models - aka “Python steps” - are standalone python scripts that use the python libraries. These are commonly used for data formatting such as the pre and post-processing steps, and are also appropriate for simple models (such as ARIMA Statsmodels). A Wallaroo Python model can be composed of one or more Python script that matches the Wallaroo requirements.
This is contrasted with Custom Models, also known as Bring Your Own Predict (BYOP) that allow for custom model inference methods with supporting scripts and artifacts. These are used with pre-trained models (PyTorch, Tensorflow, etc) along with their supporting artifacts such as other Python modules, scripts, model files, etc.
Python Models Requirements
Python scripts packaged as Python models in Wallaroo have the following requirements.
- At least one
.py
Python script file with the following:Must be compatible with Python version 3.8.
Imports the
mac.types.InferenceData
included with the Wallaroo SDK. For example:from mac.types import InferenceData
Includes the following method as the entry point for Wallaroo model inferencing:
def process_data(input_data: InferenceData) -> InferenceData: # additional code block here
Only one implementation of
process_data(input_data: InferenceData) -> InferenceData
is allowed. There can be as many Python scripts included in the .zip file as needed, but only one can have this method as the entry point.The
process_data
function must return a dictionary where the keys are strings and the values are NumPy arrays. In the case of single values (scalars) these must be single-element arrays. For example:def process_data(input_data: InferenceData) -> InferenceData: # return a dictionary with the field output that transforms the input field `variable` to its value to the 10th power. return { 'output' : np.rint(np.power(10, input_data["variable"])) }
InferenceData
represents a dictionary of numpy arrays where the first dimension is always the batch size. The type annotations set in theinput_schema
andoutput_schema
for the model when uploaded must be present and correct.process_data
accepts and returnsInferenceData
. Any other implementations will return an error.
(Optional): A
requirements.txt
file that includes any additional Python libraries required by the Python script with the following requirements:- The Python libraries must match the targeted infrastructure. For details on uploading models to a specific infrastructures such as
ARM
, see Automated Model Packaging. - The Python libraries must be compatible with Python version python==3.8.6.
- The Python libraries must match the targeted infrastructure. For details on uploading models to a specific infrastructures such as
The Python script, optional requirements.txt
file, and artifacts are packaged in a .zip
file with the Python script and optional requirements.txt
file in the root folder. For example, the sample files stored in the folder preprocess-step
:
/preprocss-step
sample-script.py
requirements.txt
/artifacts
datalist.csv
The files are packaged into a .zip
file. For example, the following packages the contents of the folder preprocess_step
into preprocess_step.zip
.
zip -r preprocess_step.zip preprocess_step/*
In the example below, the Python model is used as a pre processing step for another ML model. It accepts as an input the InferenceData
submitted as part of an inference request. It then formats the data and outputs a dictionary of numpy arrays with the field tensor
. This data is then able to be passed to the next model in a pipeline step.
import datetime
import logging
import numpy as np
import pandas as pd
import wallaroo
from mac.types import InferenceData
logger = logging.getLogger(__name__)
_vars = [
"bedrooms",
"bathrooms",
"sqft_living",
"sqft_lot",
"floors",
"waterfront",
"view",
"condition",
"grade",
"sqft_above",
"sqft_basement",
"lat",
"long",
"sqft_living15",
"sqft_lot15",
"house_age",
"renovated",
"yrs_since_reno",
]
def process_data(input_data: InferenceData) -> InferenceData:
input_df = pd.DataFrame(input_data)
thisyear = datetime.datetime.now().year
input_df["house_age"] = thisyear - input_df["yr_built"]
input_df["renovated"] = np.where((input_df["yr_renovated"] > 0), 1, 0)
input_df["yrs_since_reno"] = np.where(
input_df["renovated"],
input_df["yr_renovated"] - input_df["yr_built"],
0,
)
input_df = input_df.loc[:, _vars]
return {"tensor": input_df.to_numpy(dtype=np.float32)}
In line with other Wallaroo inference results, the outputs of a Python step that returns a pandas DataFrame or Arrow Table will be listed in the out.
metadata, with all inference outputs listed as out.{variable 1}
, out.{variable 2}
, etc. For example, a postprocessing Python step that is the final model step in a pipeline with the output field output
is included in the out
dataset as the field out.output
in the Wallaroo inference result.
time | in.tensor | out.output | anomaly.count | |
---|---|---|---|---|
0 | 2023-06-20 20:23:28.395 | [0.6878518042, 0.1760734021, -0.869514083, 0.3.. | [12.886651039123535] | 0 |
Python Libraries
Python libraries required by the included Python script are specified in the requirements.txt
file included in the .zip file. These requirements and the versions of libraries should be exactly the same between creating the model and deploying it in Wallaroo.
The requirements.txt
file specifies:
Python Libraries available through PyPi.org and the specific version. For example:
requests == 2.32.2
Python Wheels as Python model artifacts: Python Wheels as Python model artifacts are included in the .zip file and are referred to in the Python model’s
requirements.txt
file based on the relative path within the .zip file.For example, if the Python model’s .zip file includes the Python Wheel
libraries/custom_wheel.whl
├── libraries │ └── custom_wheel.whl ├── main.py └── requirements.txt
Then the
requirements.txt
file included with the Python model’s .zip file refers to this Python Wheel as:libraries/custom_wheel.whl
External Python Wheels: Python Wheels that are available from external sources (aka - not included as Python model artifacts):
- Must be referred to by the full URL.
- Must be available from the Wallaroo instance.
For example, to include the Python Wheel hosted at
https://example.wallaroo.ai/libraries/custom_wheel.whl
, therequirements.txt
file included with the Python model’s .zip file refers to this Python Wheel as:https://example.wallaroo.ai/libraries/custom_wheel.whl
Extra Index URL: For Python libraries that require the
--extra-index-url
flag:- Set the
--extra-index-url
flag with the full URL to the extra index. This must be available from the Wallaroo instance. - In the next line, specify the Python library and version.
- Repeat the steps above for each Python library with an extra index URL.
For example, to include the extra index URL
https://download.pytorch.org/whl/cu117
for thetorchvision
Python library, therequirements.txt
file included with the Python model’s .zip file refers to this Python Wheel as:--extra-index-url https://download.pytorch.org/whl/cu117 torchvision==0.15.0
- Set the
Containerized Model Runtimes
Parameter | Description |
---|---|
Web Site | https://huggingface.co/models |
Supported Libraries |
|
Frameworks | The following Hugging Face pipelines are supported by Wallaroo.
|
During the model upload process, the Wallaroo instance will attempt to convert the model to a Native Wallaroo Runtime. If unsuccessful, a Wallaroo Containerized Runtime for the model is generated instead. See the model deployment section for details on how to configure pipeline resources based on the model’s runtime.
Hugging Face Schemas
Input and output schemas for each Hugging Face pipeline are defined below. Note that adding additional inputs not specified below will raise errors, except for the following:
Framework.HUGGING_FACE_IMAGE_TO_TEXT
Framework.HUGGING_FACE_TEXT_CLASSIFICATION
Framework.HUGGING_FACE_SUMMARIZATION
Framework.HUGGING_FACE_TRANSLATION
Additional inputs added to these Hugging Face pipelines will be added as key/pair value arguments to the model’s generate method. If the argument is not required, then the model will default to the values coded in the original Hugging Face model’s source code.
See the Hugging Face Pipeline documentation for more details on each pipeline and framework.
Wallaroo Framework | Reference |
---|---|
Framework.HUGGING_FACE_FEATURE_EXTRACTION |
Schemas:
input_schema = pa.schema([
pa.field('inputs', pa.string())
])
output_schema = pa.schema([
pa.field('output', pa.list_(
pa.list_(
pa.float64(),
list_size=128
),
))
])
Wallaroo Framework | Reference |
---|---|
Framework.HUGGING_FACE_IMAGE_CLASSIFICATION |
Schemas:
input_schema = pa.schema([
pa.field('inputs', pa.list_(
pa.list_(
pa.list_(
pa.int64(),
list_size=3
),
list_size=100
),
list_size=100
)),
pa.field('top_k', pa.int64()),
])
output_schema = pa.schema([
pa.field('score', pa.list_(pa.float64(), list_size=2)),
pa.field('label', pa.list_(pa.string(), list_size=2)),
])
Wallaroo Framework | Reference |
---|---|
Framework.HUGGING_FACE_IMAGE_SEGMENTATION |
Schemas:
input_schema = pa.schema([
pa.field('inputs',
pa.list_(
pa.list_(
pa.list_(
pa.int64(),
list_size=3
),
list_size=100
),
list_size=100
)),
pa.field('threshold', pa.float64()),
pa.field('mask_threshold', pa.float64()),
pa.field('overlap_mask_area_threshold', pa.float64()),
])
output_schema = pa.schema([
pa.field('score', pa.list_(pa.float64())),
pa.field('label', pa.list_(pa.string())),
pa.field('mask',
pa.list_(
pa.list_(
pa.list_(
pa.int64(),
list_size=100
),
list_size=100
),
)),
])
Wallaroo Framework | Reference |
---|---|
Framework.HUGGING_FACE_IMAGE_TO_TEXT |
Any parameter that is not part of the required inputs
list will be forwarded to the model as a key/pair value to the underlying models generate
method. If the additional input is not supported by the model, an error will be returned.
Schemas:
input_schema = pa.schema([
pa.field('inputs', pa.list_( #required
pa.list_(
pa.list_(
pa.int64(),
list_size=3
),
list_size=100
),
list_size=100
)),
# pa.field('max_new_tokens', pa.int64()), # optional
])
output_schema = pa.schema([
pa.field('generated_text', pa.list_(pa.string())),
])
Wallaroo Framework | Reference |
---|---|
Framework.HUGGING_FACE_OBJECT_DETECTION |
Schemas:
input_schema = pa.schema([
pa.field('inputs',
pa.list_(
pa.list_(
pa.list_(
pa.int64(),
list_size=3
),
list_size=100
),
list_size=100
)),
pa.field('threshold', pa.float64()),
])
output_schema = pa.schema([
pa.field('score', pa.list_(pa.float64())),
pa.field('label', pa.list_(pa.string())),
pa.field('box',
pa.list_( # dynamic output, i.e. dynamic number of boxes per input image, each sublist contains the 4 box coordinates
pa.list_(
pa.int64(),
list_size=4
),
),
),
])
Wallaroo Framework | Reference |
---|---|
Framework.HUGGING_FACE_QUESTION_ANSWERING |
Schemas:
input_schema = pa.schema([
pa.field('question', pa.string()),
pa.field('context', pa.string()),
pa.field('top_k', pa.int64()),
pa.field('doc_stride', pa.int64()),
pa.field('max_answer_len', pa.int64()),
pa.field('max_seq_len', pa.int64()),
pa.field('max_question_len', pa.int64()),
pa.field('handle_impossible_answer', pa.bool_()),
pa.field('align_to_words', pa.bool_()),
])
output_schema = pa.schema([
pa.field('score', pa.float64()),
pa.field('start', pa.int64()),
pa.field('end', pa.int64()),
pa.field('answer', pa.string()),
])
Wallaroo Framework | Reference |
---|---|
Framework.HUGGING_FACE_STABLE_DIFFUSION_TEXT_2_IMG |
Schemas:
input_schema = pa.schema([
pa.field('prompt', pa.string()),
pa.field('height', pa.int64()),
pa.field('width', pa.int64()),
pa.field('num_inference_steps', pa.int64()), # optional
pa.field('guidance_scale', pa.float64()), # optional
pa.field('negative_prompt', pa.string()), # optional
pa.field('num_images_per_prompt', pa.string()), # optional
pa.field('eta', pa.float64()) # optional
])
output_schema = pa.schema([
pa.field('images', pa.list_(
pa.list_(
pa.list_(
pa.int64(),
list_size=3
),
list_size=128
),
list_size=128
)),
])
Wallaroo Framework | Reference |
---|---|
Framework.HUGGING_FACE_SUMMARIZATION |
Any parameter that is not part of the required inputs
list will be forwarded to the model as a key/pair value to the underlying models generate
method. If the additional input is not supported by the model, an error will be returned.
Schemas:
input_schema = pa.schema([
pa.field('inputs', pa.string()),
pa.field('return_text', pa.bool_()),
pa.field('return_tensors', pa.bool_()),
pa.field('clean_up_tokenization_spaces', pa.bool_()),
# pa.field('extra_field', pa.int64()), # every extra field you specify will be forwarded as a key/value pair
])
output_schema = pa.schema([
pa.field('summary_text', pa.string()),
])
Wallaroo Framework | Reference |
---|---|
Framework.HUGGING_FACE_TEXT_CLASSIFICATION |
Schemas
input_schema = pa.schema([
pa.field('inputs', pa.string()),
pa.field('top_k', pa.int64()),
pa.field('function_to_apply', pa.string()),
])
output_schema = pa.schema([
pa.field('label', pa.list_(pa.string(), list_size=2)), # list with a number of items same as top_k, list_size can be skipped but may lead in worse performance
pa.field('score', pa.list_(pa.float64(), list_size=2)), # list with a number of items same as top_k, list_size can be skipped but may lead in worse performance
])
Wallaroo Framework | Reference |
---|---|
Framework.HUGGING_FACE_TRANSLATION |
Any parameter that is not part of the required inputs
list will be forwarded to the model as a key/pair value to the underlying models generate
method. If the additional input is not supported by the model, an error will be returned.
Schemas:
input_schema = pa.schema([
pa.field('inputs', pa.string()), # required
pa.field('return_tensors', pa.bool_()), # optional
pa.field('return_text', pa.bool_()), # optional
pa.field('clean_up_tokenization_spaces', pa.bool_()), # optional
pa.field('src_lang', pa.string()), # optional
pa.field('tgt_lang', pa.string()), # optional
# pa.field('extra_field', pa.int64()), # every extra field you specify will be forwarded as a key/value pair
])
output_schema = pa.schema([
pa.field('translation_text', pa.string()),
])
Wallaroo Framework | Reference |
---|---|
Framework.HUGGING_FACE_ZERO_SHOT_CLASSIFICATION |
Schemas:
input_schema = pa.schema([
pa.field('inputs', pa.string()), # required
pa.field('candidate_labels', pa.list_(pa.string(), list_size=2)), # required
pa.field('hypothesis_template', pa.string()), # optional
pa.field('multi_label', pa.bool_()), # optional
])
output_schema = pa.schema([
pa.field('sequence', pa.string()),
pa.field('scores', pa.list_(pa.float64(), list_size=2)), # same as number of candidate labels, list_size can be skipped by may result in slightly worse performance
pa.field('labels', pa.list_(pa.string(), list_size=2)), # same as number of candidate labels, list_size can be skipped by may result in slightly worse performance
])
Wallaroo Framework | Reference |
---|---|
Framework.HUGGING_FACE_ZERO_SHOT_IMAGE_CLASSIFICATION |
Schemas:
input_schema = pa.schema([
pa.field('inputs', # required
pa.list_(
pa.list_(
pa.list_(
pa.int64(),
list_size=3
),
list_size=100
),
list_size=100
)),
pa.field('candidate_labels', pa.list_(pa.string(), list_size=2)), # required
pa.field('hypothesis_template', pa.string()), # optional
])
output_schema = pa.schema([
pa.field('score', pa.list_(pa.float64(), list_size=2)), # same as number of candidate labels
pa.field('label', pa.list_(pa.string(), list_size=2)), # same as number of candidate labels
])
Wallaroo Framework | Reference |
---|---|
Framework.HUGGING_FACE_ZERO_SHOT_OBJECT_DETECTION |
Schemas:
input_schema = pa.schema([
pa.field('images',
pa.list_(
pa.list_(
pa.list_(
pa.int64(),
list_size=3
),
list_size=640
),
list_size=480
)),
pa.field('candidate_labels', pa.list_(pa.string(), list_size=3)),
pa.field('threshold', pa.float64()),
# pa.field('top_k', pa.int64()), # we want the model to return exactly the number of predictions, we shouldn't specify this
])
output_schema = pa.schema([
pa.field('score', pa.list_(pa.float64())), # variable output, depending on detected objects
pa.field('label', pa.list_(pa.string())), # variable output, depending on detected objects
pa.field('box',
pa.list_( # dynamic output, i.e. dynamic number of boxes per input image, each sublist contains the 4 box coordinates
pa.list_(
pa.int64(),
list_size=4
),
),
),
])
Wallaroo Framework | Reference |
---|---|
Framework.HUGGING_FACE_SENTIMENT_ANALYSIS | Hugging Face Sentiment Analysis |
Wallaroo Framework | Reference |
---|---|
Framework.HUGGING_FACE_TEXT_GENERATION |
Any parameter that is not part of the required inputs
list will be forwarded to the model as a key/pair value to the underlying models generate
method. If the additional input is not supported by the model, an error will be returned.
input_schema = pa.schema([
pa.field('inputs', pa.string()),
pa.field('return_tensors', pa.bool_()), # optional
pa.field('return_text', pa.bool_()), # optional
pa.field('return_full_text', pa.bool_()), # optional
pa.field('clean_up_tokenization_spaces', pa.bool_()), # optional
pa.field('prefix', pa.string()), # optional
pa.field('handle_long_generation', pa.string()), # optional
# pa.field('extra_field', pa.int64()), # every extra field you specify will be forwarded as a key/value pair
])
output_schema = pa.schema([
pa.field('generated_text', pa.list_(pa.string(), list_size=1))
])
Wallaroo Framework | Reference |
---|---|
Framework.HUGGING_FACE_AUTOMATIC_SPEECH_RECOGNITION |
Sample input and output schema.
input_schema = pa.schema([
pa.field('inputs', pa.list_(pa.float32())), # required: the audio stored in numpy arrays of shape (num_samples,) and data type `float32`
pa.field('return_timestamps', pa.string()) # optional: return start & end times for each predicted chunk
])
output_schema = pa.schema([
pa.field('text', pa.string()), # required: the output text corresponding to the audio input
pa.field('chunks', pa.list_(pa.struct([('text', pa.string()), ('timestamp', pa.list_(pa.float32()))]))), # required (if `return_timestamps` is set), start & end times for each predicted chunk
])
Parameter | Description |
---|---|
Web Site | https://pytorch.org/ |
Supported Libraries |
|
Framework | Framework.PYTORCH aka pytorch |
Supported File Types | pt ot pth in TorchScript format |
IMPORTANT NOTE
The PyTorch model must be in TorchScript format. scripting (i.e.torch.jit.script()
is always recommended over tracing (i.e. torch.jit.trace()
).From the PyTorch documentation: “Scripting preserves dynamic control flow and is valid for inputs of different sizes.”
For more details, see TorchScript-based ONNX Exporter: Tracing vs Scripting.
During the model upload process, Wallaroo optimizes models by converting them to the Wallaroo Native Runtime, if possible, or running the model directly in the Wallaroo Containerized Runtime. See the Model Deploy for details on how to configure pipeline resources based on the model’s runtime.
- IMPORTANT CONFIGURATION NOTE: For PyTorch input schemas, the floats must be
pyarrow.float32()
for the PyTorch model to be converted to the Native Wallaroo Runtime during the upload process.
PyTorch Input and Output Schemas
PyTorch input and output schemas have additional requirements depending on whether the PyTorch model is single input/output or multiple input/output. This refers to the number of columns:
- Single Input/Output: Has one input and one output column.
- Multiple Input/Output: Has more than one input or more than one output column.
The column names for the model can be anything. For example:
- Model Input Fields:
length
width
intensity
etc
When creating the input and output schemas for uploading a PyTorch model in Wallaroo, the field names must match the following requirements. For example, for multi-column PyTorch models, the input would be:
- Data Schema Input Fields:
input_1
input_2
input_3
input_...
For single input/output PyTorch model, the field names must be input
and output
. For example, if the input field is a List of Floats of size 10, and the output field is a list of floats of list size one, the input and output schemas are:
input_schema = pa.schema([
pa.field('input', pa.list_(pa.float32(), list_size=10))
])
output_schema = pa.schema([
pa.field('output', pa.list_(pa.float32(), list_size=1))
])
For multi input/output PyTorch models, the data schemas for each input and output field must be named input_1, input_2...
and output_1
, output_2
, etc. These must be in the same order that the PyTorch model is trained to accept them.
For example, a multi input/output PyTorch model that takes the following inputs and outputs:
- Inputs
input_1
: List of Floats of length 10.input_2
: List of Floats of length 5.
- Outputs
output_1
: List of Floats of length 3.output_2
: List of Floats of length 2.
The following input and output schemas would be used.
input_schema = pa.schema([
pa.field('input_1', pa.list_(pa.float32(), list_size=10)),
pa.field('input_2', pa.list_(pa.float32(), list_size=5))
])
output_schema = pa.schema([
pa.field('output_1', pa.list_(pa.float32(), list_size=3)),
pa.field('output_2', pa.list_(pa.float32(), list_size=2))
])
Sci-kit Learn aka SKLearn.
Parameter | Description |
---|---|
Web Site | https://scikit-learn.org/stable/index.html |
Supported Libraries |
|
Framework | Framework.SKLEARN aka sklearn |
During the model upload process, Wallaroo optimizes models by converting them to the Wallaroo Native Runtime, if possible, or running the model directly in the Wallaroo Containerized Runtime. See the Model Deploy for details on how to configure pipeline resources based on the model’s runtime.
SKLearn Schema Inputs
SKLearn schema follows a different format than other models. To prevent inputs from being out of order, the inputs should be submitted in a single row in the order the model is trained to accept, with all of the data types being the same. For example, the following DataFrame has 4 columns, each column a float
.
sepal length (cm) | sepal width (cm) | petal length (cm) | petal width (cm) | |
---|---|---|---|---|
0 | 5.1 | 3.5 | 1.4 | 0.2 |
1 | 4.9 | 3.0 | 1.4 | 0.2 |
For submission to an SKLearn model, the data input schema will be a single array with 4 float values.
input_schema = pa.schema([
pa.field('inputs', pa.list_(pa.float64(), list_size=4))
])
When submitting as an inference, the DataFrame is converted to rows with the column data expressed as a single array. The data must be in the same order as the model expects, which is why the data is submitted as a single array rather than JSON labeled columns: this insures that the data is submitted in the exact order as the model is trained to accept.
Original DataFrame:
sepal length (cm) | sepal width (cm) | petal length (cm) | petal width (cm) | |
---|---|---|---|---|
0 | 5.1 | 3.5 | 1.4 | 0.2 |
1 | 4.9 | 3.0 | 1.4 | 0.2 |
Converted DataFrame:
inputs | |
---|---|
0 | [5.1, 3.5, 1.4, 0.2] |
1 | [4.9, 3.0, 1.4, 0.2] |
SKLearn Schema Outputs
Outputs for SKLearn that are meant to be predictions
or probabilities
when output by the model are labeled in the output schema for the model when uploaded to Wallaroo. For example, a model that outputs either 1 or 0 as its output would have the output schema as follows:
output_schema = pa.schema([
pa.field('predictions', pa.int32())
])
When used in Wallaroo, the inference result is contained in the out
metadata as out.predictions
.
pipeline.infer(dataframe)
time | in.inputs | out.predictions | anomaly.count | |
---|---|---|---|---|
0 | 2023-07-05 15:11:29.776 | [5.1, 3.5, 1.4, 0.2] | 0 | 0 |
1 | 2023-07-05 15:11:29.776 | [4.9, 3.0, 1.4, 0.2] | 0 | 0 |
Parameter | Description |
---|---|
Web Site | https://www.tensorflow.org/api_docs/python/tf/keras/Model |
Supported Libraries |
|
Framework | Framework.KERAS aka keras |
Supported File Types | SavedModel format as .zip file and HDF5 format |
During the model upload process, Wallaroo optimizes models by converting them to the Wallaroo Native Runtime, if possible, or running the model directly in the Wallaroo Containerized Runtime. See the Model Deploy for details on how to configure pipeline resources based on the model’s runtime.
TensorFlow Keras SavedModel Format
TensorFlow Keras SavedModel models are .zip file of the SavedModel format. For example, the Aloha sample TensorFlow model is stored in the directory alohacnnlstm
:
├── saved_model.pb
└── variables
├── variables.data-00000-of-00002
├── variables.data-00001-of-00002
└── variables.index
This is compressed into the .zip file alohacnnlstm.zip
with the following command:
zip -r alohacnnlstm.zip alohacnnlstm/
See the SavedModel guide for full details.
TensorFlow Keras H5 Format
Wallaroo supports the H5 for Tensorflow Keras models.
Parameter | Description |
---|---|
Web Site | https://xgboost.ai/ |
Supported Libraries |
|
Framework | Framework.XGBOOST aka xgboost |
Supported File Types | pickle (XGB files are not supported.) |
During the model upload process, Wallaroo optimizes models by converting them to the Wallaroo Native Runtime, if possible, or running the model directly in the Wallaroo Containerized Runtime. See the Model Deploy for details on how to configure pipeline resources based on the model’s runtime.
Since the Wallaroo 2024.1 release, XGBoost support is enhanced to performantly support a wider set of XGBoost models. XGBoost models are not required to be trained with ONNX nomenclature in order to successfully convert to a performant runtime.
XGBoost Types Support
The following XGBoost model types are supported by Wallaroo. XGBoost models not supported by Wallaroo are supported via the Custom Models, also known as Bring Your Own Predict (BYOP).
XGBoost Model Type | Wallaroo Packaging Supported |
---|---|
XGBClassifier | √ |
XGBRegressor | √ |
Booster Classifier | √ |
Booster Classifier | √ |
Booster Regressor | √ |
Booster Random Forest Regressor | √ |
Booster Random Forest Classifier | √ |
XGBRFClassifier | √ |
XGBRFRegressor | √ |
XGBRanker* | X |
- XGBRanker XGBoost models are currently supported via converting them to BYOP models.
XGBoost Schema Inputs
XGBoost schema follows a different format than other models. To prevent inputs from being out of order, the inputs should be submitted in a single row in the order the model is trained to accept, with all of the data types being the same. If a model is originally trained to accept inputs of different data types, it will need to be retrained to only accept one data type for each column - typically pa.float64()
is a good choice.
For example, the following DataFrame has 4 columns, each column a float
.
sepal length (cm) | sepal width (cm) | petal length (cm) | petal width (cm) | |
---|---|---|---|---|
0 | 5.1 | 3.5 | 1.4 | 0.2 |
1 | 4.9 | 3.0 | 1.4 | 0.2 |
For submission to an XGBoost model, the data input schema will be a single array with 4 float values.
input_schema = pa.schema([
pa.field('inputs', pa.list_(pa.float32(), list_size=4))
])
When submitting as an inference, the DataFrame is converted to rows with the column data expressed as a single array. The data must be in the same order as the model expects, which is why the data is submitted as a single array rather than JSON labeled columns: this insures that the data is submitted in the exact order as the model is trained to accept.
Original DataFrame:
sepal length (cm) | sepal width (cm) | petal length (cm) | petal width (cm) | |
---|---|---|---|---|
0 | 5.1 | 3.5 | 1.4 | 0.2 |
1 | 4.9 | 3.0 | 1.4 | 0.2 |
Converted DataFrame:
inputs | |
---|---|
0 | [5.1, 3.5, 1.4, 0.2] |
1 | [4.9, 3.0, 1.4, 0.2] |
XGBoost Schema Outputs
Outputs for XGBoost are labeled based on the trained model outputs.
Outputs for XBoost that are meant to be predictions
or probabilities
must be labeled as part of the output schema. For example, a model that outputs either 1 or 0 as its output would have the output schema as follows:
output_schema = pa.schema([
pa.field('predictions', pa.float32()),
])
When used in Wallaroo, the inference result is contained in the out
metadata as out.predictions
.
pipeline.infer(dataframe)
time | in.inputs | out.predictions | anomaly.count | |
---|---|---|---|---|
0 | 2023-07-05 15:11:29.776 | [5.1, 3.5, 1.4, 0.2] | 0 | 0 |
1 | 2023-07-05 15:11:29.776 | [4.9, 3.0, 1.4, 0.2] | 0 | 0 |
Parameter | Description |
---|---|
Web Site | https://www.python.org/ |
Supported Libraries | python==3.8 |
Framework | Framework.CUSTOM aka custom |
Custom Models, also known as Bring Your Own Predict (BYOP) allow for custom model inference methods with supporting scripts and artifacts. These are used with pre-trained models (PyTorch, Tensorflow, etc) along with their supporting artifacts such as other Python modules, scripts, model files, etc.
Contrast this with Wallaroo Python models - aka “Python steps” - are standalone python scripts that use the python libraries. These are commonly used for data formatting such as the pre and post-processing steps, and are also appropriate for simple models (such as ARIMA Statsmodels). A Wallaroo Python model can be composed of one or more Python script that matches the Wallaroo requirements.
Custom Model File Requirements
Custom Model (BYOP) models are uploaded to Wallaroo via a ZIP file with the following components:
Artifact | Type | Description |
---|---|---|
Python scripts aka .py files with classes that extend mac.inference.Inference and mac.inference.creation.InferenceBuilder | Python Script | Extend the classes mac.inference.Inference and mac.inference.creation.InferenceBuilder . These are included with the Wallaroo SDK. Further details are in Custom Model Script Requirements. Note that there is no specified naming requirements for the classes that extend mac.inference.Inference and mac.inference.creation.InferenceBuilder - any qualified class name is sufficient as long as these two classes are extended as defined below. |
requirements.txt | Python requirements file | This sets the Python libraries used for the Wallaroo Custom Model model. These libraries should be targeted for Python 3.8 compliance. These requirements and the versions of libraries should be exactly the same between creating the model and deploying it in Wallaroo. This insures that the script and methods will function exactly the same as during the model creation process. |
Other artifacts | Files | Other models, files, and other artifacts used in support of this model. |
For example, the if the Wallaroo Custom Model model will be known as vgg_clustering
, the contents may be in the following structure, with vgg_clustering
as the storage directory:
vgg_clustering\
feature_extractor.h5
kmeans.pkl
custom_inference.py
requirements.txt
Note the inclusion of the custom_inference.py
file. This file name is not required - any Python script or scripts that extend the classes listed above are sufficient. This Python script could have been named vgg_custom_model.py
or any other name as long as it includes the extension of the classes listed above.
The sample Wallaroo Custom Model model file is created with the command zip -r vgg_clustering.zip vgg_clustering/
.
Wallaroo Custom Model uses the Wallaroo SDK mac
module, included in the Wallaroo SDK 2023.2.1 and above. See the Wallaroo SDK Install Guides for instructions on installing the Wallaroo SDK.
Custom Model Script Requirements
The entry point of the Wallaroo Custom Model model is any python script that extends the following classes. These are included with the Wallaroo SDK. The required methods that must be overridden are specified in each section below.
mac.inference.Inference
interface serves model inferences based on submitted input some input. Its purpose is to serve inferences for any supported arbitrary model framework (e.g.scikit
,keras
etc.).
mac.inference.creation.InferenceBuilder
builds a concreteInference
, i.e. instantiates anInference
object, loads the appropriate model and assigns the model to to the Inference object.
mac.inference.Inference
mac.inference.Inference Objects
Object | Type | Description |
---|---|---|
model (Required) | [Any] | One or more objects that match the expected_model_types . This can be a ML Model (for inference use), a string (for data conversion), etc. See Custom Model Examples for examples. |
mac.inference.Inference Methods
Method | Returns | Description |
---|---|---|
expected_model_types (Required) | Set | Returns a Set of models expected for the inference as defined by the developer. Typically this is a set of one. Wallaroo checks the expected model types to verify that the model submitted through the InferenceBuilder method matches what this Inference class expects. |
_predict (input_data: mac.types.InferenceData) (Required) | mac.types.InferenceData | The entry point for the Wallaroo inference with the following input and output parameters that are defined when the model is updated.
InferenceDataValidationError exception is raised when the input data does not match mac.types.InferenceData . |
raise_error_if_model_is_not_assigned | N/A | Error when a model is not set to Inference . |
raise_error_if_model_is_wrong_type | N/A | Error when the model does not match the expected_model_types . |
IMPORTANT NOTE
Verify that the inputs and outputs match theInferenceData
input and output types: a Dictionary of numpy arrays defined by the input_schema
and output_schema
parameters when uploading the model to the Wallaroo instance. The following code is an example of a Dictionary of numpy arrays.preds = self.model.predict(data)
preds = preds.numpy()
rows, _ = preds.shape
preds = preds.reshape((rows,))
return {"prediction": preds} # a Dictionary of numpy arrays.
The example, the expected_model_types
can be defined for the KMeans
model.
from sklearn.cluster import KMeans
class SampleClass(mac.inference.Inference):
@property
def expected_model_types(self) -> Set[Any]:
return {KMeans}
mac.inference.creation.InferenceBuilder
InferenceBuilder
builds a concrete Inference
, i.e. instantiates an Inference
object, loads the appropriate model and assigns the model to the Inference.
Each model that is included requires its own InferenceBuilder
. InferenceBuilder
loads one model, then submits it to the Inference
class when created. The Inference
class checks this class against its expected_model_types()
Set.
mac.inference.creation.InferenceBuilder Methods
Method | Returns | Description |
---|---|---|
create(config mac.config.inference.CustomInferenceConfig) (Required) | The custom Inference instance. | Creates an Inference subclass, then assigns a model and attributes. The CustomInferenceConfig is used to retrieve the config.model_path , which is a pathlib.Path object pointing to the folder where the model artifacts are saved. Every artifact loaded must be relative to config.model_path . This is set when the Wallaroo Custom Model .zip file is uploaded and the environment for running it in Wallaroo is set. For example: loading the artifact vgg_clustering\feature_extractor.h5 would be set with config.model_path \ feature_extractor.h5 . The model loaded must match an existing module. For our example, this is from sklearn.cluster import KMeans , and this must match the Inference expected_model_types . |
inference | custom Inference instance. | Returns the instantiated custom Inference object created from the create method. |
Python Libraries
Python libraries required by the included Python script are specified in the requirements.txt
file included in the .zip file. These requirements and the versions of libraries should be exactly the same between creating the model and deploying it in Wallaroo.
The requirements.txt
file specifies:
Python Libraries available through PyPi.org and the specific version. For example:
requests == 2.32.2
Python Wheels as BYOP artifacts: Python Wheels as BYOP artifacts are included in the .zip file and are referred to in the BYOP’s
requirements.txt
file based on the relative path within the .zip file.For example, if the BYOP .zip file includes the Python Wheel
libraries/custom_wheel.whl
├── libraries │ └── custom_wheel.whl ├── main.py └── requirements.txt
Then the
requirements.txt
file included with the BYOP’s .zip file refers to this Python Wheel as:libraries/custom_wheel.whl
External Python Wheels: Python Wheels that are available from external sources (aka - not included as BYOP artifacts):
- Must be referred to by the full URL.
- Must be available from the Wallaroo instance.
For example, to include the Python Wheel hosted at
https://example.wallaroo.ai/libraries/custom_wheel.whl
, therequirements.txt
file included with the BYOP’s .zip file refers to this Python Wheel as:https://example.wallaroo.ai/libraries/custom_wheel.whl
Extra Index URL: For Python libraries that require the
--extra-index-url
flag:- Set the
--extra-index-url
flag with the full URL to the extra index. This must be available from the Wallaroo instance. - In the next line, specify the Python library and version.
- Repeat the steps above for each Python library with an extra index URL.
For example, to include the extra index URL
https://download.pytorch.org/whl/cu117
for thetorchvision
Python library, therequirements.txt
file included with the BYOP’s .zip file refers to this Python Wheel as:--extra-index-url https://download.pytorch.org/whl/cu117 torchvision==0.15.0
- Set the
Custom Model Runtime
Custom Model always run in the containerized model runtime.
Custom Model Inputs
Custom Model inputs are defined during model upload in Apache Arrow Schema format with the following conditions:
- By default, data inputs are optional unless they are specified with
nullable=False
. - The arbitrary Python code must be aware of the optional and required fields and how to manage those inputs.
- Specific Data Types conditions:
- Scaler: Scaler values can be
Null
. - Lists: Lists must either be empty
[]
or an an array ofNull
values, for example[None]
, but cannot be passed asNull
outside of an array.
- Scaler: Scaler values can be
- By default, columns with only the
None
orNull
value are assigned by Python asNullArray
, which is an array with all values ofNull
. In these situations, the schema must be specified.
Custom Model Inputs Example
The following code sample demonstrates managing optional inputs.
The arbitrary Python code has three inputs:
input_1
: A required List of floats.input_2
: An optional List of floats.multiply_factor
: An optional scaler float.
The following demonstrates setting the input and output schemas when uploading the sample code to Wallaroo.
import wallaroo
import pyarrow as pa
input_schema = pa.schema([
pa.field('input_1', pa.list_(pa.float32()), nullable=False), # fields are optional by default unless `nullable` is set to `False`
pa.field('input_2', pa.list_(pa.float32())),
pa.field('multiply_factor', pa.int32()),
])
output_schema = pa.schema([
pa.field('output', pa.list_(pa.float32())),
])
The following demonstrates different valid inputs based on the input schemas. These fields are submitted either as a pandas DataFrame or an Apache Arrow table when submitted for inference requests.
Note that each time the data is translated to an Apache Arrow table, the input schema is specified so the accurate data types are assigned to the column, even with the column values are Null
or None
.
The following input has all fields and values translated into an Apache Arrow table, then submitted as an inference request to a pipeline with our sample BYOP model.
input_1 = [[1., 2.], [3., 4.]]
input_2 = [[5., 6.], [7., 8.]]
multiply_factor = [2, 3]
arrow_table = pa.table({"input_1": input_1, "input_2": input_2, "multiply_factor": multiply_factor}, schema=input_schema)
display(arrow_)table
input_1 = [[1., 2.], [3., 4.]]
input_2 = [[], []]
multiply_factor = [None, None]
arrow_table = pa.table({"input_1": input_1, "input_2": input_2, "multiply_factor": multiply_factor}, schema=input_schema)
arrow_table
pipeline.infer(arrow_table)
pyarrow.Table
time: timestamp[ms]
in.input_1: list<item: float> not null
child 0, item: float
in.input_2: list<item: float> not null
child 0, item: float
in.multiply_factor: int32 not null
out.output: list<item: double> not null
child 0, item: double
anomaly.count: uint32 not null
----
time: [[2024-04-30 09:12:01.445,2024-04-30 09:12:01.445]]
in.input_1: [[[1,2],[3,4]]]
in.input_2: [[[5,6],[7,8]]]
in.multiply_factor: [[2,3]]
out.output: [[[12,16],[30,36]]]
anomaly.count: [[0,0]]
In the following example input_2
has two empty lists, stored into a pandas DataFrame and submitted for the inference request.
dataframe = pd.DataFrame({'input_1': [[1., 2.], [3., 4.]], 'input_2': [[], []], 'multiply_factor': [2, 3]})
display(dataframe)
input_1 | input_2 | multiply_factor | |
---|---|---|---|
0 | [1.0, 2.0] | [] | 2 |
1 | [3.0, 4.0] | [] | 3 |
For the following example, input_2
is an empty list, with multiply_factor
set to None
. This is stored in an Apache Arrow table for the inference request.
input_1 = [[1., 2.], [3., 4.]]
input_2 = [[], []]
multiply_factor = [None, None]
arrow_table = pa.table({"input_1": input_1, "input_2": input_2, "multiply_factor": multiply_factor}, schema=input_schema)
display(arrow_table)
pyarrow.Table
input_1: list<item: float> not null
child 0, item: float
input_2: list<item: float>
child 0, item: float
multiply_factor: int32
----
input_1: [[[1,2],[3,4]]]
input_2: [[[],[]]]
multiply_factor: [[null,null]]
pipeline.infer(arrow_table)
pyarrow.Table
time: timestamp[ms]
in.input_1: list<item: float> not null
child 0, item: float
in.input_2: list<item: float> not null
child 0, item: float
in.multiply_factor: int32 not null
out.output: list<item: double> not null
child 0, item: double
anomaly.count: uint32 not null
----
time: [[2024-04-30 09:07:42.467,2024-04-30 09:07:42.467]]
in.input_1: [[[1,2],[3,4]]]
in.input_2: [[[],[]]]
in.multiply_factor: [[null,null]]
out.output: [[[1,2],[3,4]]]
anomaly.count: [[0,0]]
Parameter | Description |
---|---|
Web Site | https://mlflow.org |
Supported Libraries | mlflow==1.30.0 |
For models that do not fall under the supported model frameworks, organizations can use containerized MLFlow ML Models.
This guide details how to add ML Models from a model registry service into Wallaroo.
Wallaroo supports both public and private containerized model registries. See the Wallaroo Private Containerized Model Container Registry Guide for details on how to configure a Wallaroo instance with a private model registry.
Wallaroo users can register their trained MLFlow ML Models from a containerized model container registry into their Wallaroo instance and perform inferences with it through a Wallaroo pipeline.
As of this time, Wallaroo only supports MLFlow 1.30.0 containerized models. For information on how to containerize an MLFlow model, see the MLFlow Documentation.
Data Schemas
ONNX models deployed to Wallaroo have the following data requirements.
- Equal rows constraint: The number of input rows and output rows must match.
- All inputs are tensors: The inputs are tensor arrays with the same shape.
- Data Type Consistency: Data types within each tensor are of the same type.
Equal Rows Constraint
Inference performed through ONNX models are assumed to be in batch format, where each input row corresponds to an output row. This is reflected in the in
fields returned for an inference. In the following example, each input row for an inference is related directly to the inference output.
df = pd.read_json('./data/cc_data_1k.df.json')
display(df.head())
result = ccfraud_pipeline.infer(df.head())
display(result)
INPUT
tensor | |
---|---|
0 | [-1.0603297501, 2.3544967095000002, -3.5638788326, 5.1387348926, -1.2308457019, -0.7687824608, -3.5881228109, 1.8880837663, -3.2789674274, -3.9563254554, 4.0993439118, -5.6539176395, -0.8775733373, -9.131571192000001, -0.6093537873, -3.7480276773, -5.0309125017, -0.8748149526000001, 1.9870535692, 0.7005485718000001, 0.9204422758, -0.1041491809, 0.3229564351, -0.7418141657, 0.0384120159, 1.0993439146, 1.2603409756, -0.1466244739, -1.4463212439] |
1 | [-1.0603297501, 2.3544967095000002, -3.5638788326, 5.1387348926, -1.2308457019, -0.7687824608, -3.5881228109, 1.8880837663, -3.2789674274, -3.9563254554, 4.0993439118, -5.6539176395, -0.8775733373, -9.131571192000001, -0.6093537873, -3.7480276773, -5.0309125017, -0.8748149526000001, 1.9870535692, 0.7005485718000001, 0.9204422758, -0.1041491809, 0.3229564351, -0.7418141657, 0.0384120159, 1.0993439146, 1.2603409756, -0.1466244739, -1.4463212439] |
2 | [-1.0603297501, 2.3544967095000002, -3.5638788326, 5.1387348926, -1.2308457019, -0.7687824608, -3.5881228109, 1.8880837663, -3.2789674274, -3.9563254554, 4.0993439118, -5.6539176395, -0.8775733373, -9.131571192000001, -0.6093537873, -3.7480276773, -5.0309125017, -0.8748149526000001, 1.9870535692, 0.7005485718000001, 0.9204422758, -0.1041491809, 0.3229564351, -0.7418141657, 0.0384120159, 1.0993439146, 1.2603409756, -0.1466244739, -1.4463212439] |
3 | [-1.0603297501, 2.3544967095000002, -3.5638788326, 5.1387348926, -1.2308457019, -0.7687824608, -3.5881228109, 1.8880837663, -3.2789674274, -3.9563254554, 4.0993439118, -5.6539176395, -0.8775733373, -9.131571192000001, -0.6093537873, -3.7480276773, -5.0309125017, -0.8748149526000001, 1.9870535692, 0.7005485718000001, 0.9204422758, -0.1041491809, 0.3229564351, -0.7418141657, 0.0384120159, 1.0993439146, 1.2603409756, -0.1466244739, -1.4463212439] |
4 | [0.5817662108, 0.09788155100000001, 0.1546819424, 0.4754101949, -0.19788623060000002, -0.45043448540000003, 0.016654044700000002, -0.0256070551, 0.0920561602, -0.2783917153, 0.059329944100000004, -0.0196585416, -0.4225083157, -0.12175388770000001, 1.5473094894000001, 0.2391622864, 0.3553974881, -0.7685165301, -0.7000849355000001, -0.1190043285, -0.3450517133, -1.1065114108, 0.2523411195, 0.0209441826, 0.2199267436, 0.2540689265, -0.0450225094, 0.10867738980000001, 0.2547179311] |
OUTPUT
time | in.tensor | out.dense_1 | anomaly.count | |
---|---|---|---|---|
0 | 2023-11-17 20:34:17.005 | [-1.0603297501, 2.3544967095, -3.5638788326, 5.1387348926, -1.2308457019, -0.7687824608, -3.5881228109, 1.8880837663, -3.2789674274, -3.9563254554, 4.0993439118, -5.6539176395, -0.8775733373, -9.131571192, -0.6093537873, -3.7480276773, -5.0309125017, -0.8748149526, 1.9870535692, 0.7005485718, 0.9204422758, -0.1041491809, 0.3229564351, -0.7418141657, 0.0384120159, 1.0993439146, 1.2603409756, -0.1466244739, -1.4463212439] | [0.99300325] | 0 |
1 | 2023-11-17 20:34:17.005 | [-1.0603297501, 2.3544967095, -3.5638788326, 5.1387348926, -1.2308457019, -0.7687824608, -3.5881228109, 1.8880837663, -3.2789674274, -3.9563254554, 4.0993439118, -5.6539176395, -0.8775733373, -9.131571192, -0.6093537873, -3.7480276773, -5.0309125017, -0.8748149526, 1.9870535692, 0.7005485718, 0.9204422758, -0.1041491809, 0.3229564351, -0.7418141657, 0.0384120159, 1.0993439146, 1.2603409756, -0.1466244739, -1.4463212439] | [0.99300325] | 0 |
2 | 2023-11-17 20:34:17.005 | [-1.0603297501, 2.3544967095, -3.5638788326, 5.1387348926, -1.2308457019, -0.7687824608, -3.5881228109, 1.8880837663, -3.2789674274, -3.9563254554, 4.0993439118, -5.6539176395, -0.8775733373, -9.131571192, -0.6093537873, -3.7480276773, -5.0309125017, -0.8748149526, 1.9870535692, 0.7005485718, 0.9204422758, -0.1041491809, 0.3229564351, -0.7418141657, 0.0384120159, 1.0993439146, 1.2603409756, -0.1466244739, -1.4463212439] | [0.99300325] | 0 |
3 | 2023-11-17 20:34:17.005 | [-1.0603297501, 2.3544967095, -3.5638788326, 5.1387348926, -1.2308457019, -0.7687824608, -3.5881228109, 1.8880837663, -3.2789674274, -3.9563254554, 4.0993439118, -5.6539176395, -0.8775733373, -9.131571192, -0.6093537873, -3.7480276773, -5.0309125017, -0.8748149526, 1.9870535692, 0.7005485718, 0.9204422758, -0.1041491809, 0.3229564351, -0.7418141657, 0.0384120159, 1.0993439146, 1.2603409756, -0.1466244739, -1.4463212439] | [0.99300325] | 0 |
4 | 2023-11-17 20:34:17.005 | [0.5817662108, 0.097881551, 0.1546819424, 0.4754101949, -0.1978862306, -0.4504344854, 0.0166540447, -0.0256070551, 0.0920561602, -0.2783917153, 0.0593299441, -0.0196585416, -0.4225083157, -0.1217538877, 1.5473094894, 0.2391622864, 0.3553974881, -0.7685165301, -0.7000849355, -0.1190043285, -0.3450517133, -1.1065114108, 0.2523411195, 0.0209441826, 0.2199267436, 0.2540689265, -0.0450225094, 0.1086773898, 0.2547179311] | [0.0010916889] | 0 |
All Inputs Are Tensors
All inputs into an ONNX model must be tensors. This requires that the shape of each element is the same. For example, the following is a proper input:
t [
[2.35, 5.75],
[3.72, 8.55],
[5.55, 97.2]
]
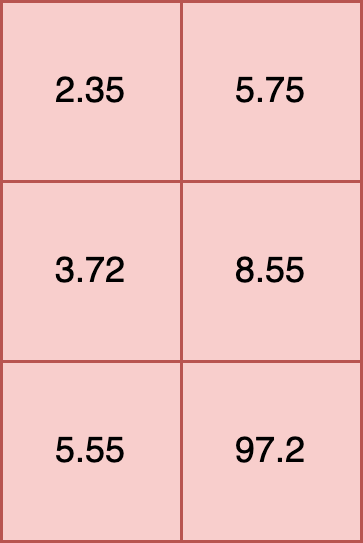
Another example is a 2,2,3 tensor, where the shape of each element is (3,), and each element has 2 rows.
t = [
[2.35, 5.75, 19.2],
[3.72, 8.55, 10.5]
],
[
[5.55, 7.2, 15.7],
[9.6, 8.2, 2.3]
]
In this example each element has a shape of (2,)
. Tensors with elements of different shapes, known as ragged tensors, are not supported. For example:
t = [
[2.35, 5.75],
[3.72, 8.55, 10.5],
[5.55, 97.2]
])
**INVALID SHAPE**
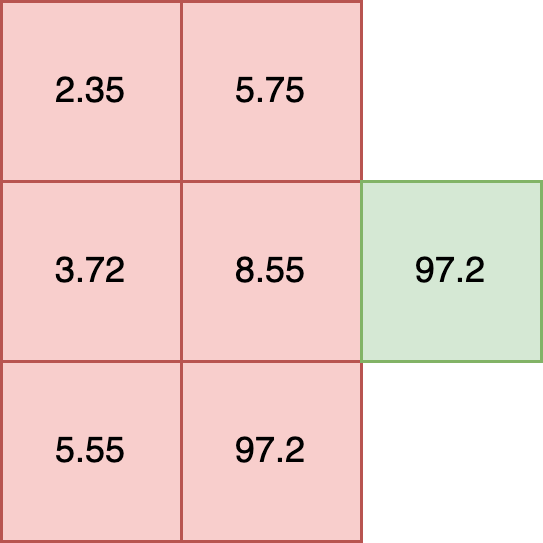
For models that require ragged tensor or other shapes, see other data formatting options such as Bring Your Own Predict models.
Data Type Consistency
All inputs into an ONNX model must have the same internal data type. For example, the following is valid because all of the data types within each element are float32
.
t = [
[2.35, 5.75],
[3.72, 8.55],
[5.55, 97.2]
]
The following is invalid, as it mixes floats and strings in each element:
t = [
[2.35, "Bob"],
[3.72, "Nancy"],
[5.55, "Wani"]
]
The following inputs are valid, as each data type is consistent within the elements.
df = pd.DataFrame({
"t": [
[2.35, 5.75, 19.2],
[5.55, 7.2, 15.7],
],
"s": [
["Bob", "Nancy", "Wani"],
["Jason", "Rita", "Phoebe"]
]
})
df
t | s | |
---|---|---|
0 | [2.35, 5.75, 19.2] | [Bob, Nancy, Wani] |
1 | [5.55, 7.2, 15.7] | [Jason, Rita, Phoebe] |
Models can be converted to native runtime models by meeting the following requirements.
Wallaroo ONNX Requirements
Wallaroo natively supports Open Neural Network Exchange (ONNX) models into the Wallaroo engine.
Parameter | Description |
---|---|
Web Site | https://onnx.ai/ |
Supported Libraries | See table below. |
Framework | Framework.ONNX aka onnx |
Runtime | Native aka onnx |
Supported Versions | 1.12.1 |
The following ONNX versions models are supported:
ONNX Version | ONNX IR Version | ONNX OPset Version | ONNX ML Opset Version |
---|---|---|---|
1.12.1 | 8 | 17 | 3 |
- If converting another ML Model to ONNX (PyTorch, XGBoost, etc) using the onnxconverter-common library, the supported
DEFAULT_OPSET_NUMBER
is 17.
Using different versions or settings outside of these specifications may result in inference issues and other unexpected behavior.
ONNX models always run in the Wallaroo Native Runtime space.
The following ML Model versions and Python libraries are supported by Wallaroo. When using the Wallaroo autoconversion library or working with a local version of the Wallaroo SDK, use the following versions for maximum compatibility.
Library | Supported Version |
---|---|
Python | 3.8.6 and above |
Wallaroo | wallaroo==2024.3.3 |
onnx | onnx==1.12.0 |
tensorflow | tensorflow==2.9.3 |
keras | keras==2.9.0 |
pytorch | torch==2.0.0 |
sk-learn aka scikit-learn | scikit-learn==1.3.0 |
statsmodels | statsmodels==0.13.2 |
XGBoost | xgboost==1.7.4 |
MLFlow | mlflow==1.30.0 |
Supported Data Types
The following data types are supported for transporting data to and from Wallaroo in the following run times:
- ONNX
- TensorFlow
- MLFlow
Data Type Conditions
The following conditions apply to data types used in inference requests.
None
orNull
data types are not submitted. All fields must have submitted values that match their data type. For example, if the schema expects afloat
value, then some value of typefloat
must be submitted and can not beNone
orNull
. If a schema expects a string value, then some value of type string must be submitted, etc. The exception are BYOP models, which can accept optional inputs.datetime
data types must be converted tostring
.- ONNX models support multiple inputs only of the same data type.
Runtime | BFloat16* | Float16 | Float32 | Float64 |
---|---|---|---|---|
ONNX | X | X | ||
TensorFlow | X | X | X | |
MLFlow | X | X | X |
* (Brain Float 16, represented internally as a f32)
Runtime | Int8 | Int16 | Int32 | Int64 |
---|---|---|---|---|
ONNX | X | X | X | X |
TensorFlow | X | X | X | X |
MLFlow | X | X | X | X |
Runtime | Uint8 | Uint16 | Uint32 | Uint64 |
---|---|---|---|---|
ONNX | X | X | X | X |
TensorFlow | X | X | X | X |
MLFlow | X | X | X | X |
Runtime | Boolean | Utf8 (String) | Complex 64 | Complex 128 | FixedSizeList* |
---|---|---|---|---|---|
ONNX | X | ||||
Tensor | X | X | X | ||
MLFlow | X | X | X |
* Fixed sized lists of any of the previously supported data types.
Can I Import My Own Notebooks Into Wallaroo?
Yes! Jupyter Hub is provided as a service in your Wallaroo instance. So feel free to import your notebooks, models, and data to get right to work.
What Is The Difference Between A Model And A Pipeline In Wallaroo?
A Model, or Machine Learning Model (ML), has been trained by data scientists to take in data and return some result. A Wallaroo Pipeline can set one or more models as steps in the pipeline. This lets you submit data to a pipeline, have that data sent to each model, then return a result.
This provides you with the power to set the order for how pipelines provide inferences in different orders, chain different models together to run comparisons, or any other combination of tasks you can come up with.
Can I Train A Model In Wallaroo?
While Wallaroo contains an entire Python library and allows you full access and control within that environment, models should be trained outside of Wallaroo, then imported into Wallaroo to be deployed and run as an object in the Wallaroo engine.
How Can I Serve My Models Up To Return Predictions?
Once you have uploaded your models to Wallaroo and deployed a pipeline that steps through how information is submitted to each model you use for the inference, you can serve your models in the following ways:
- Run an Inference from a File : Submit a file with an array of values, and return the results derived from them.
- Run an Inference through a Pipeline Deployment URL: Submit your inference request through a HTTP Pipeline Deployment URL created when the pipeline is deployed.
Can I A/B Test My Models In Wallaroo?
Yes! Wallaroo supports A/B testing through the following mechanisms:
- Set up Control and Challenger models
- Define a pipeline to split data between the two models
- Test the Challenger model and return the results.
- Perform a Shadow Deploy to send data to both models, but only return to the user the results from the Control model. This allows users to test Challenger models without sacrificing production deployments.
Where Can I See Pipeline Performance Metrics?
Pipeline metrics can be seen through the Wallaroo Dashboard through the following process:
- From the Wallaroo Dashboard, set the current workspace from the top left dropdown list.
- Select View Pipelines from the pipeline’s row.
- To view details on the pipeline, select the name of the pipeline.
- A list of the pipeline’s details will be displayed.
- Select Metrics to view the following information. From here you can select the time period to display metrics from through the drop down to display the following:
- Requests per second
- Cluster inference rate
- Inference latency
- The Audit Log and Anomaly Log are available to view further details of the pipeline’s activities.
How Are Wallaroo Enterprise And Wallaroo Community Edition Different?
Wallaroo Community is different from Wallaroo Enterprise based on the available features and restrictions.
Feature | Wallaroo Community | Wallaroo Enterprise |
---|---|---|
Max Number of Cores | 32 | Unlimited |
Max Number of Users | 2 | Unlimited |
Max Number of Deployed Pipelines | 2 | Unlimited |
Max Steps per Pipeline | 5 | Unlimited |
Single Sign On | ⃠ | √ |
Compute Auto-Scaling | ⃠ | √ |
Wallaroo Support Services | Wallaroo Community Slack | Wallaroo Enterprise Support |